AI Policy
AI Policy: Building Trust in the Age of Intelligent Systems
Artificial intelligence (AI) is transforming how we live, perform tasks, and interact. Ranging from automated content generation to autonomous vehicles, artificial intelligence is no longer only a buzzword—it’s a main force in the digital revolution. However, as this technology improves, the significance of applying a well-planned AI policy becomes important.
In this guide, we will cover what an AI policy actually is, why it’s necessary, how both governments and organizations are approaching it, and how businesses—mainly those in high-compute segments like GPU4HOST, GPU server providers, and AI businesses—can get a good advantage from setting up robust AI policies.
About AI Policy
It is basically a legal framework or set of instructions engineered to guide the development, deployment, and utilization of AI-based technologies. It makes sure that AI is utilized ethically and in alignment with organizational or national norms. AI policies can change in scope—from mentioning all internal uses in companies to highlighting national tactics for AI governance.
This policy mainly addresses:
- Responsibility structures
- Legal AI-based development
- Data privacy and safety
- Transparency & explainability
- Compliance & regulation
- Bias and fairness mitigation
Why AI Policy Is Necessary
With AI-based systems impacting essential decisions in healthcare, finance, hiring, and law enforcement, the requirement for oversight is crucial. AI, while robust, can also boost biases, create data security hazards, and result in unexpected outcomes. That’s where an AI Policy plays an essential role in ensuring accountable development and deployment.
For organizations in high-performance computing (HPC), like all those deploying GPU servers, GPU clusters, or using NVIDIA A100 infrastructure, policies help describe secure limits, operational guidelines, and technical compliance standards.
AI Policy Areas to Consider
At the time of developing an internal or governmental AI Policy, it’s necessary to consider many AI policy areas, consisting of:
- Algorithmic Transparency: Making sure that AI-based models can be easily interpreted and defined.
- Data Management Policies: What data is gathered, how it’s kept, and how it’s utilized.
- Security Standards: Mainly necessary for businesses utilizing a GPU dedicated server for high-level data processing.
- Bias and Clear Audits: Constant evaluations to ensure equity in results.
- Human Mistake: Maintaining human responsibility in decision-making procedures.
AI Policy Templates for Businesses
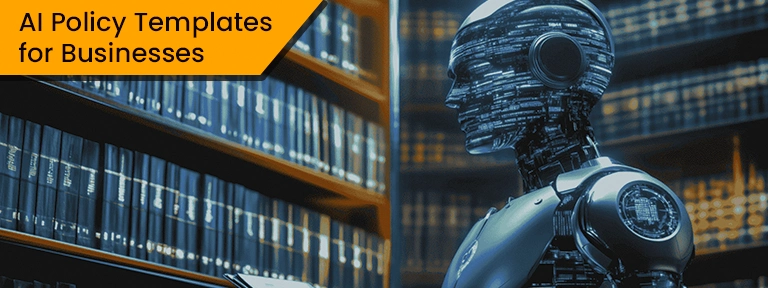
To rationalize adoption, several companies utilize AI policy templates. These documents give an appropriate standard for creating personalized internal instructions. A powerful AI policy template should consist of:
- Incident reply mechanisms
- Purpose & scope of the AI policy
- Roles & responsibilities
- Compliance & enforcement
- Data governance standards
- Descriptions and key terms
The Role of the Center for AI Policy
The Center for AI Policy is an organization that emphasizes on creating public policy suggestions for ethical and secure AI. It gets together with policymakers, experts, and industry stakeholders to direct how AI should be regulated. Their work generally shapes worldwide and national AI-based frameworks and informs company-based AI policies.
Organizations and developers opting to align with global measures should follow valuable insights and instructions published by such policy think tanks.
Challenges in Applying AI Policies
Despite its significance, developing and imposing an AI Policy isn’t without challenges:
- Shortage of technical understanding: Decision-makers may sometimes struggle to understand AI challenges.
- Progressing regulatory landscape: With AI quickly growing, policies can rapidly become outdated.
- Integration with IT infrastructure: For organizations utilizing challenging systems such as GPU clusters, integrating policy imposition mechanisms needs technical expertise.
- Balancing both innovation and control: Excessive regulation can smother innovation, mainly in new business and R&D environments.
Case Study: AI Policy in the GPU Infrastructure Segment
Let’s think about a hypothetical situation of any XYZ company offering high-performance GPU dedicated servers for artificial intelligence and deep learning workloads.
Situation: A customer utilizes the company’s infrastructure to create an AI-based model for facial recognition.
AI Policy Enforcement consists of:
- Checking that the data used is legally sourced and adheres to privacy guidelines.
- Making sure that model training is bias-audited.
- Deploying models only after clarity checks.
- Logging every single activity on the NVIDIA A100 servers for liability.
This planned approach reduces risks and gains users’ trust.
NSFW AI Apps & Policy Reply
Another interesting topic gaining attention is the Character AI NSFW policy. As users communicate with artificial intelligence for storytelling or complex simulation, limits need to be described regarding the right content. Platforms are now applying AI policies that forbid or restrict NSFW (Not Safe for Work) content, which leads to discussions around moderation, user liberty, and security.
A solid AI Policy should clearly specify content utilization guidelines and have technical filters to ensure adherence—mainly necessary for all those platforms using GPU servers for generative AI.
Government-Level AI Policies
All countries globally are racing to set up national AI policies that meet economic, ethical, and geopolitical aims. For example:
- The EU AI Act: Presents risk-powered classifications and bans some specific uses of AI.
- US AI Bill of Rights: Suggests guiding concepts for safeguarding citizens.
- India’s AI Mission: Centered on inclusive AI innovation and powerful infrastructure.
Enterprises utilizing AI at scale, mainly those running robust infrastructures such as NVIDIA A100-powered GPU clusters, must guarantee compliance with those guidelines across all regions they work in.
How GPU Infrastructure Supports AI Policy Compliance
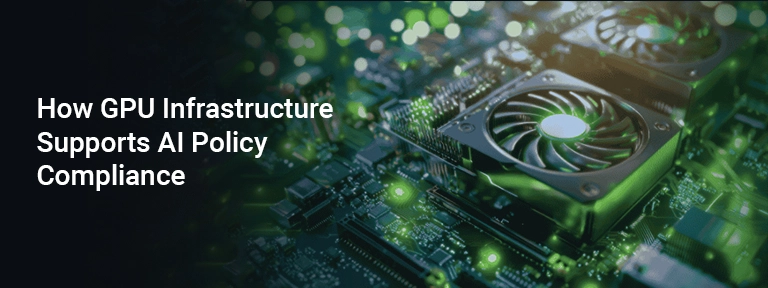
Applying a solid AI Policy needs computing infrastructure that easily supports transparency, logging, advanced model retraining, and actual monitoring. This is the case where GPU servers and GPU clusters play their remarkable part. Service providers such as GPU4HOST offer the required power and scalability to:
- Train clearer, bias-decreased models
- Run encrypted settings
- Apply safe data pipelines
- Provide sandboxed testing for sensitive apps
Conclusion
A productive AI Policy isn’t only a document—it’s a planned asset that drives innovation, compliance, and users’ trust. Even if you are a national policymaker, a founder, or a GPU server provider, it’s the right time to check policy as essential to your AI-based model development lifecycle.
By covering main AI policy areas, utilizing scalable AI policy templates, knowing about the global regulatory climate with the help of institutions such as the Center for AI Policy, and implementing policy through modern infrastructure like a GPU dedicated servers, organizations can innovate correctly and sustainably.
Frequently Asked Questions
- What are the AI policy areas?
AI policy areas consist of data security, algorithmic transparency, legal AI usage, bias mitigation, responsibility, AI safety, and intellectual property rights.
- What is AI policy?
AI policy refers to the guidelines and frameworks that guide the development, deployment, and utilization of AI-based technologies in a legal way.
- What are the components of good AI policy?
A robust AI policy has ethical instructions, data security standards, transparency guidelines, accountability structures, risk management, and constant review processes.
- How to create an AI policy?
Begin by describing AI uses, consult both ethical and legal measures, highlight clear responsibilities, set up data governance standards, and constantly update the policy as per growing tech and rules.
- How does DeepSeek’s privacy policy compare to other AI companies?
DeepSeek’s privacy policy focuses on data transparency and user control, meeting with industry leaders, though details may change in data retention, third-party sharing, etc.