Best GPU for Deep Learning, Dedicated GPU Server Hosting
GPUs can provide necessary speedups over traditional CPUs in the case of training deep neural networks. We offer bare GPU metal servers that are specially developed for carrying out all AI-based tasks. We have the best GPU for deep learning to perform AI training, inference, and many more.
Get Started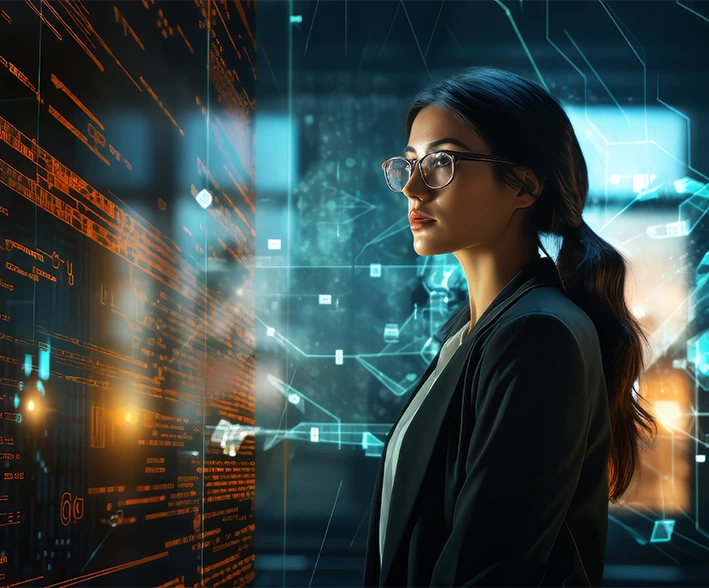
Several Reasons to Choose Our Deep Learning Server
GPU4HOST has robust GPU dedicated server hosting qualities on raw bare metal hardware, served according to demand. No more inadequacy, and many more. Experience exceptional performance, reliability, and flexibility with the help of our deep learning server, built to boost your heavy workloads seamlessly.

99.9% Uptime Guarantee
With organization-based infrastructure and data centers, GPU4HOST offers a 99.9% uptime guarantee for all hosted GPU servers for various tasks as well as neural networks.

Intel Xeon CPU
Intel Xeon has powerful processing speed and computing power, appropriate for running different programs. So you can easily utilize our Intel-Xeon-powered GPUs.

DDoS Protection
Resources among various users are completely isolated to maintain data privacy. GPU4Host defends against DDoS attacks while offering organic traffic of hosted GPUs.

SSD-Based Drives
You can never face any issue with our excellent GPU-dedicated servers for PyTorch, fully loaded with the modern Intel Xeon processors, 128 GB of RAM per server, and many more.

Dedicated IP
One of the most beneficial features is the fully dedicated IP address. Whether the affordable GPU dedicated hosting plan is completely packed with IPv6 and IPv4 Internet protocols.

Root/Admin Access
With proper admin/root access, you will be fully capable of taking complete control of your dedicated GPU servers, especially in the case of deep learning, very effortlessly and rapidly.
Budget-Friendly Pricing Plans for Deep Learning

RTX 2060
$ 269.00/month
$50.99
- Dual 10-Core E5-2660v2
- 128GB RAM
- 960GB SSD
- 1Gbps Port Speed
- GPU: Nvidia GeForce RTX 2060
- Microarchitecture: Ampere
- Max GPUs: 2
- CUDA Cores: 1920
- Tensor Cores: 240
- GPU Memory: 6GB GDDR6
- FP32 Performance: 6.5 TFLOPS
- OS: Windows / Linux
- Fully managed

RTX 4090
$ 455.00/month
$50.99
- Enterprise GPU - RTX 4090
- 256GB RAM
- 2TB NVMe + 8TB SATA
- 1Gbps Port Speed
- GPU: GeForce RTX 4090
- Microarchitecture: Ada Lovelace
- Max GPUs: 1
- CUDA Cores: 16,384
- Tensor Cores: 512
- GPU Memory: 24 GB GDDR6X
- FP32 Performance: 82.6 TFLOPS
- OS: Windows / Linux
- Fully managed

Enterprise GPU - A100
$ 869.00/month
$50.99
- 256GB RAM
- 2TB NVMe + 8TB SATA
- 1Gbps Port Speed
- OS: Windows / Linux
- GPU: Nvidia A100
- Microarchitecture: Ampere
- Max GPUs: 1
- CUDA Cores: 6912
- Tensor Cores: 432
- GPU Memory: 40GB HBM2e
- FP32 Performance: 19.5 TFLOPS
- Fully managed

V100
$ 669.00/month
$50.99
- Multi-GPU - 3xV100
- 256GB RAM
- 2TB NVMe + 8TB SATA
- 1Gbps Port Speed
- GPU: 3 x Nvidia V100
- Microarchitecture: Volta
- Max GPUs: 3
- CUDA Cores: 5,120
- Tensor Cores: 640
- GPU Memory: 16GB HBM2
- FP32 Performance: 14 TFLOPS
- OS: Windows / Linux
- Fully managed

Multiple GPU - 4xA100
$ 2,619.00/month
$50.99
- Dual 22-Core E5-2699v4
- 512GB RAM
- 4TB NVMe
- 1Gbps Port Speed
- GPU: 4xNvidia A100
- Microarchitecture: Ampere
- Max GPUs: 4
- CUDA Cores: 6912
- Tensor Cores: 432
- GPU Memory: 40GB HBM2e
- FP32 Performance: 19.5 TFLOPS
- OS: Windows / Linux
- Fully managed

Multiple GPU - 3xV100
$ 719.00/month
$50.99
- Dual 18-Core E5-2697v4
- 256GB RAM
- 2TB NVMe
- 1Gbps Port Speed
- GPU: 3xNvidia V100
- Microarchitecture: Volta
- Max GPUs: 3
- CUDA Cores: 5,120
- Tensor Cores: 640
- GPU Memory: 16GB HBM2
- FP32 Performance: 14 TFLOPS
- OS: Windows / Linux
- Fully managed
- Instant Support
- Quick Deploy
- Robust Security
How to Select the Best Dedicated GPU
Server Hosting for Deep Learning
When you are selecting GPU servers, then some factors should be kept in mind.


RT Core
RT Cores are types of accelerator units that are completely dedicated to performing real-time ray tracing with full productivity.


Tensor Cores
Tensor cores allow complex computing, powerfully accepting calculations to enhance outcomes while maintaining accuracy.


Performance
Deep learning uses the maximum floating-point of the graphics card and the maximum arithmetic computing power.


Budget Price
We provide various budget-friendly GPUs, so you can simply discover a plan that matches your requirements within your budget.


Memory Bandwidth
GPU memory bandwidth is a kind of density of the speed of data transfer among any particular system and a GPU over a bus.


Memory Capacity
Huge memory size can easily decrease the time taken to read all important information as well as decrease latency.
Right to Make a Customized Deep Learning Environment
The various well-known frameworks and tools are fully system-friendly, so please always choose the most appropriate version to download. We are glad to help you.
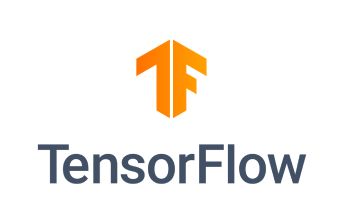
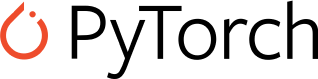
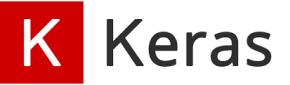
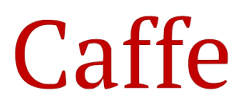
data.
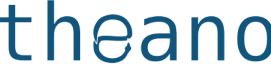
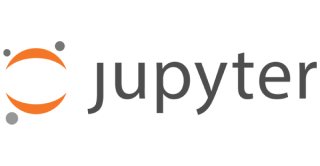
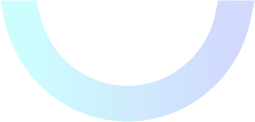

Have Any Queries?
Contact us either through a phone call or live chat and get the solution to your query.
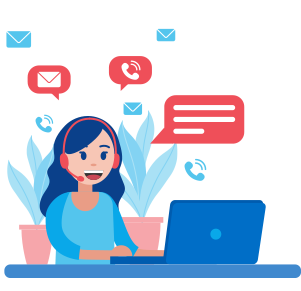